High-resolution modeling and machine learning
Machine learning, foundation models and subgrid-scale physics
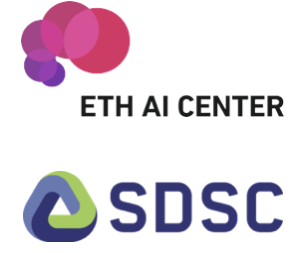
Collaboration with the Swiss Data Science Center and ETH AI Centre
Many of the subgrid-scale physics in weather and climate models are key sources of error for the representation of clouds, precipitation and circulating systems in the atmosphere. While for some of the subgrid-scale physics can in principale be resolved, but only over small domains and short time periods, for others the underlying equations are unknown. As data-driven weather forecasting has proven to be good at different lead times, we are also exploring how machine learning can improve weather forecasts from hours to weeks and months.
Projects
- external pageSwiss AIcall_made, under the auspices of the ETH AI Centre, aims to develop an open foundation climate system model with fine-tuning capabilities down to a few kilometres over the greater Alpine region. external pageFanny Lehmanncall_made will join the initiative as a postdoc and external pageLevi Lingschcall_made as a PhD student, both in collaboration with SAM.
- In a joint project with the external pageSwiss Data Science Centercall_made (SDSC), we explore ways to build physics-regulated machine learning subgrid-scale parameterizations that leverage existing accurate but computational expensive parameterizations. Our target model is ICON, which will be at the heart of the knew exascale platform (EXCLAIM) envisioned by the Center for Climate Systems Modelling (C2SM) and its partners via an OpenETH grant. In this project ICON external pagehas been coupled to an ML parameterizationcall_made developed in Python.
- In a collaboration with the ETH AI Center, we explore ways how explainability method of trained neural networks can help to explain the physics underlying regional climate patterns. Interested in an AI Ph.D. or postdoc fellowship? Please get in contact with us (before applying).
Publications
- The preprint of the first project related publication "Revisiting Machine Learning Approaches for Short- and Longwave Radiation Inference in Weather and Climate Models, Part II: Online Performance" is now under review and available for download from external pageESS Open Archive.call_made
- This is the first time that external pageICONcall_made has not only been coupled to an ML parameterization developed in Python, but both run in tandem on GPUs.
- This is the first time that external pageICONcall_made has not only been coupled to an ML parameterization developed in Python, but both run in tandem on GPUs.
- The preprint of the first project related publication "Revisiting Machine Learning Approaches for Short- and Longwave Radiation Inference in Weather and Climate Models, Part I: Offline Performance" is now under review and available for download from external pageESS Open Archive.call_made
Conferences and other acitivies
- The team presented their work at the external page2022 EMS Annual Meetingcall_made, Bonn, Germany (04/09/2022 – 09/09/2022). [external pageSessionOSA1.9call_made]
- Guillaume Bertoli presented his work on building a physics-constrained radiation scheme at the external pageCentre Henri Lebesguecall_made workshop in external pageMoulin Mer, Logonna-Daoulas, Finistère, Francecall_made
- In HS2020 and again HS2021, a team of student participating in the Data Science Lab explore whether ML method are able to predict cyclone tracks.
- Guillaume Bertoli started to explore how ML algorithms can emulate the radiation code in ICON. Guillaume graduated with a Ph.D. in external pagenumerical analysiscall_made at the departement of mathematics at the University of Geneva and previously worked on strang splitting and time stepping methods [external pageSuperconvergence of the Strang splitting when using the Crank-Nicolson schemecall_made][external pageStrang splitting method for semilinear parabolic problemscall_made].
- Kenza Amara started here Ph.D. at the AI center on explainbility.