Weather Ensemble Member Generation
In the latent spaces of Deep Neural Networks (DNN)
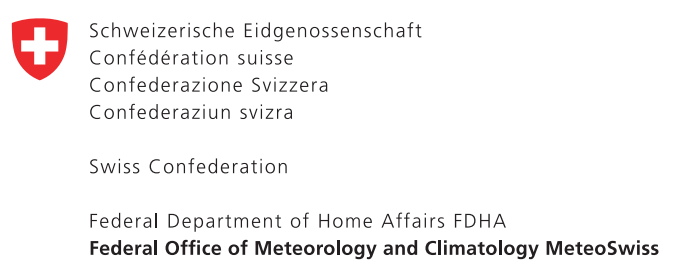
By harnessing the power of machine learning (ML), researchers from IAC in collaboration with MeteoSwiss are exploring innovative ways to improve weather forecasting and anticipate extreme events in our ever-changing climate. ML methods use representations in latent spaces, such as graph-like meshes or frequency space, to directly connect information across different scales. This approach bypasses the need for costly numerical integration and offers not only a potential speed boost but also inspires new research on tasks like ensemble member generation, inverse learning of physical model parameters, and the introduction of physical constraints.
We're investigating the potential of deep neural networks for weather model ensemble inflation, which involves generating additional ensemble members at a relatively low cost. Training data include the external pageCOSMO-1/2call_made archive and external pageNvidia's FourCastNetcall_made is used generate ensemble forecasts. To verify the performance of our ML models, we'll use observation-based verification methods typically employed for numerical weather prediction models.
By examining these aspects, our research project will contribute to a better understanding of the potential of ML-based weather model ensemble predictions. Our ultimate goal is to improve the accuracy of weather forecasts and better anticipate extreme weather events in a changing climate.
News
- Simon Adamov started his Ph.D. on deep learning for weather ensemble member generation. [Apr 2023]